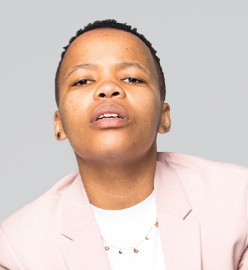
Yoliswa Mkhize, researcher, CSIR
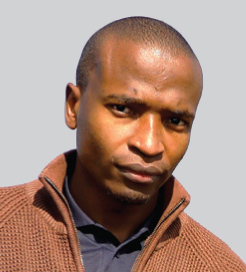
Dr Sabelo Madonsela, researcher, CSIR
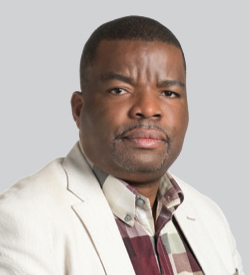
Prof Abel Ramoelo, executive director: Earth Observation Programme – South African National Space Agency (SANSA); extraordinary professor: Geography, Geoinfor-matics and Meteorology, University of Pretoria
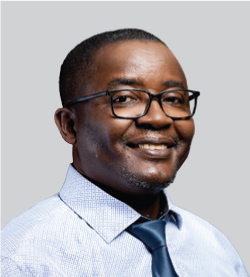
Prof Moses Azong Cho, remote sensing scientist, chief researcher & research group leader: Precision Agriculture, CSIR
Weeds pose a significant threat to crops by competing for water, nutrients, and sunlight, which can lead to reduced yields and in some cases complete crop failure. Early weed detection and control are therefore essential for sustainable farming.
This aspect of precision agriculture, known as precision weed management, can be enhanced using drone or satellite imaging. Drone or satellite imaging for weed detection has been shown to be a more cost-effective alternative to manual field scouting.
In this article, we report on a pioneering demonstration of satellite imaging for weed detection by the Council for Scientific and Industrial Research (CSIR) in Gauteng.
Method and results
On-the-ground weed data were collected simultaneously with satellite overpasses from the European Space Agency’s Sentinel-2 during the early growth stages of maize at five locations in Gauteng. Computer machine learning models were trained to correlate weed presence with Sentinel-2 image properties. Once the models achieved satisfactory training and validation results, they were applied to the entire image to detect weeds across all fields.
We achieved an accuracy rate of over 85% in detecting weeds in maize fields, significantly enhancing detection precision compared to traditional methods. These results highlight the effectiveness of Sentinel-2 imagery in distinguishing the spectral properties of maize from those of weeds, particularly in relation to differences in biomass and canopy structure. However, spectral models require seasonal retraining with ground-truth data to account for spatial and temporal variations in weed growth.
To address this challenge, we explored the use of the canopy leaf density, also known as the leaf area index (LAI), for distinguishing between weed-free and weed-infested plots during the early growth stages of maize. This approach achieved an accuracy of 92% in detecting weeds at early growth stages, demonstrating its potential for improving weed management strategies.
Conclusions
The findings from our research are being shared through the CSIR’s Precision Agriculture Information System (PAIS), an online platform that allows producers to access weed detection maps and other decision-support tools. By making data readily available, PAIS promotes the adoption of precision agriculture practices, helping producers improve efficiency, reduce costs, and enhance sustainability.
As satellite or drone remote sensing and machine learning technologies advance, weed detection systems will become even more accurate and efficient. Integrating these tools with automated machinery, such as precision sprayers, will enable more targeted herbicide application, minimising chemical use and environmental impact. Continued investment in research, along with producer training, will be key to maximising the benefits of these innovations for sustainable agriculture.
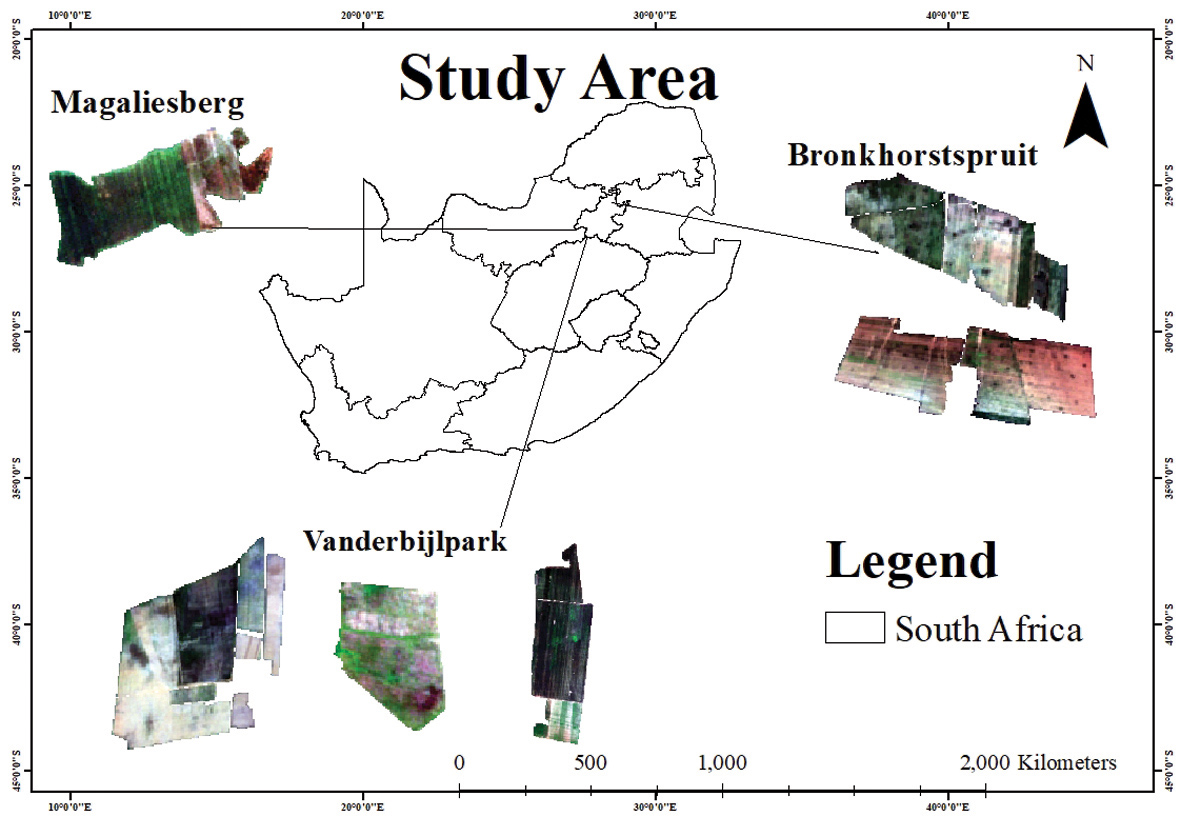
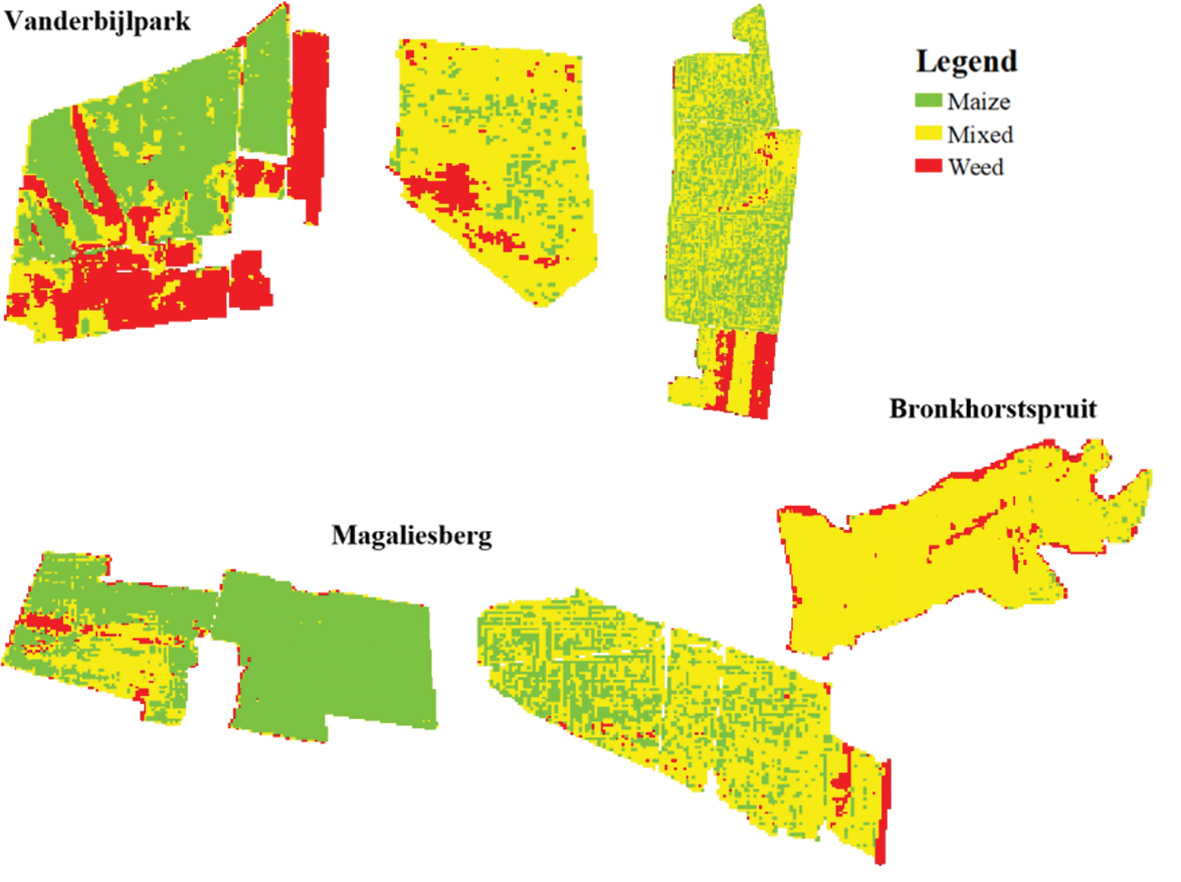