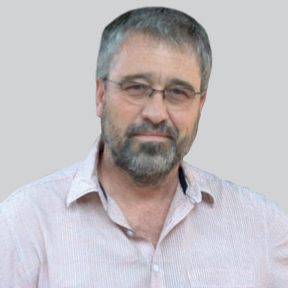
der Westhuizen,
SA Stud Book
The information revolution brought about by the internet – and with it amazingly accurate and fast search engines – has resulted in the accumulation of knowledge unsurpassed by anything before. What have been hailed in livestock breeding as good observations and conclusions in the past, are now either proven, rejected, or even put to better use in breeding programmes.
Past research
The forefront focus during the 1960s to 1980s was on functionality of farm animals, their anatomy and physiological processes that underpinned suitability in specific environmental and production systems. It was also the era known to be the onset of computer database developments and with those, objective measurements and recordings of economically important traits. It was the dawn of big data and the proper use of mathematical models to predict the genetic merit of candidates for selection of parents of the new generation production animals.
Although the theory of mixed model methodology to calculate best linear unbiased prediction (BLUP) breeding values was already developed, the practical application thereof had to wait for computing power to catch up. This mainly happened in the late 1980s and early 1990s before it could be put to full use. The very effective and accurate genetic merit predictions, relying on breeders to accurately record parentage and objectively measured traits (within well-defined contemporary groups), immediately caused a new set of challenges.
Effective genetic selection without well-defined long-term breeding objectives, especially in its simple form that favours only a few traits, mainly led to strict within family selection and high inbreeding of progeny and, in many cases, unfavourable correlated responses for other economically important traits. The traits that mostly suffered from these practices are female fitness traits, such as reproduction and survivability (or sometimes referred to as ‘involuntary culling’). Again, although the theories associated with the setting up and application of breeding and selection objectives were well established, the combination thereof with BLUP breeding values only started taking off in this century when scientists and breeders realised the damages caused by single trait selection in all its forms.
This more balanced approach and carefully constructed mating programmes assisted scientists and informed breeders to achieve breeding goals that favour animals with the preferred genetic merit to produce offspring that will excel in being more profitable, given the environmental constraints, production system and market requirements. Such programmes also look beyond just the next generation and ensure maintaining longer-term genetic diversity and therefore future selection opportunities.
Traditionally scientists with higher levels of speciality in animal breeding and genetics focused on either of the sciences underpinning molecular or quantitative genetics. Scientific advances in quantitative genetics led to the development of practical ways to predict genetic merit of farm animals, mainly based on generalised knowledge and assumptions regarding additive gene actions. In simple terms, thereby ‘adding’ the relatively small effect of numerous genes contributing towards the merit of each potential breeding bull (or ram) and heifer (or ewe) as a selection candidate.
In the meantime, after the successful sequencing of the human genome and followed by many other species, including bovine (cattle), ovine (sheep) and other farm animals, the race was on to discover the location of individual genes contributing towards genetic differences in performance for economically important traits. Although many of these traits of economic importance and influence have been discovered, it soon dawned that molecular means alone cannot identify every gene. Interactions on the whole genome is also not known.
The development of the bead chip that enables a clear view of differences among the genomes of individual animals came as a gamechanger. Those currently in use will reveal in the order of 50 000 (SNP) base pairs from around 3,5 billion (thousand million) in the genome of most farm animals. Initially researchers used the information from a (fairly large) number of animals in a population (breed) with high accurate BLUP breeding values, to link this information to an animal’s genetic merit. This became known as the single nucleotide polymorphism (SNP) effects, or direct genomic values (DGVs), fairly similar to multiple genes, each with a very small effect.
Although these predictions assist in attaining genetic merit for young or unrecorded animals (like in the case of sex-limited traits, only measurable on one of the sexes) the application thereof requires a large investment in such a reference population. It also still needs a second step of combining the DGVs with the BLUP estimated breeding values (EBVs) resulting in genomic breeding values (GEBVs). This became known as the two-step (or multi-step).
Traditional BLUP breeding values need two main sources of information from breeders, namely pedigree information and recordings of the traits of importance. Pedigree information is needed because related animals share common parts (genes) on their genomes. Due to the random nature of inheritance of parts of the chromosomes carrying the genetic code, the true genetic relationships (except for parent-offspring relationships) are based on average assumptions. (It is ‘assumed’ that you are 25% related to each of you grandparents and 50% related to your brother or sister, but these percentages depend on the random samples passed on and can therefore vary between 0% and 100% with the highest likelihood near the assumed percentage.)
By genotyping strategically in a population, covering as many as possible unrelated animals and those of high impact (many progeny), genomic information can be combined with traditional BLUP breeding values to increase the accuracy of prediction for young and other unrecorded animals. The true genetic relationship therefore replaces the assumed value when a BLUP breeding value prediction is carried out. This is also known as ‘single-step’ genomic breeding values of gBLUP.
The inclusion of genomic information and applying single-step gBLUP methodology can now be deemed as common practice in leading countries.
Current research
Current research focuses on, among other things:
- Addressing the contribution of and impact on changes in global climatic change
Researchers find marked genetic differences among ruminants in methane emissions, even at the same production levels. The complex relationship between the ruminant species and types of microorganisms in the rumens of individuals and the genetic control by the ‘host’ (the animal itself) is the focus of many research projects. Exciting findings create possibilities to select for ruminants with lower methane outputs whilst they are at the same time more efficient in utilising the available feed resources. As most breeding programmes also make use of genomic information of the breeding animals, the micro biome is also now genotyped in these research programmes.Farm animals are also subjected to changes in climatic conditions. Some animals cope better than others and can reproduce, produce, and regain their ability to thrive when climatic conditions return to acceptable parameters. There are, therefore, new possibilities to select breeding stock with more resilient offspring. These animals will be able to ensure that production animals can be bred that will be able to perform extremely well when the climatic conditions allow for it, but will keep on producing even in adverse conditions.New breeding objectives also focus on lifetime profitability versus short-term profit when selection candidates are considered.
- Automation and the era of big data
Technological advances, especially electronic sensors and similar devices, are also affecting the ability to accelerate the rate and accuracy of recording important events and properties in livestock. Recording of behaviour, weight changes, physiological state, movement and location and other properties are more and more automated and uploaded onto centralised computer servers, or even used in calculations on the spot for management and other interventions.Smart systems also use algorithms linked to artificial intelligence and deep machine learning to assist. Early detection of disease, metabolic disturbance or conditions, coming on heat and other events are becoming commonplace in some systems. The value of intelligent bio-informatic systems is also becoming more important as the large volumes of data still need to be changed to useful information.
- Diagnostic tests with bigger value
Rapid results from diagnostic and other informative tests making use of body tissue and milk samples also hold big promise. Traditionally, the near infra-red laser spectrum was used for milk samples to determine milk solids percentage (fat, protein, and lactose), somatic cells and milk urea nitrogen. By using the mid infra-red spectrum and new regression formulas, much more information can be obtained from a milk sample. The most important information in milk samples shows the metabolic state of the cow and can be used to avoid ketosis and acidosis and even identify cows utilising protein best from pastures (best converters) whilst emitting lower levels of methane. Similarly, the use of rapid pregnancy and mastitis tests is also taking the world by storm.
For livestock breeders of South Africa, one of the biggest challenges is still to be able to keep up with the latest developments influencing economically sustainable livestock production. South African producers are always challenged to be competitive – locally and on a global scale. Current services to predict the genetic merit of suitable next-generation parents and to optimise mating programmes are available from a service provider like SA Stud Book. Basic accurate recording is always a prerequisite for any breeding programme and to manage herds and flocks optimally. This is the starting point before considering any other new developments.