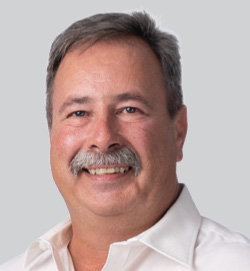
Ag solutions manager, CNH Industrial
As the global population continues to rise, the agricultural sector faces significant challenges in meeting the increasing demand for food, while also addressing issues such as resource scarcity, climate change, and labour shortages. However, advancements in artificial intelligence (AI) have brought about a new era in agriculture, transforming traditional farming methods and enabling producers to optimise production, improve sustainability, and enhance decision-making.
Precision farming and predictive analytics
AI technologies, such as machine learning algorithms and predictive analytics, play a crucial role in precision farming. By analysing vast amounts of data collected from various sources, including weather patterns, soil conditions, crop health, historical and live machine metrics and historical yield data, AI algorithms can provide valuable insights and recommendations to producers. This enables them to make data-driven decisions related to planting, irrigation, fertilisation, and pest control, leading to optimised resource allocation, reduced costs, and increased yields. In some instances, AI technology is built into agricultural equipment, providing real-time analytics which optimises the current function and activity of the machinery.
Raven’s OMNiDRIVE™ technology brings advanced autonomous capabilities to your grain collection and transport needs. You can request an autonomous grain cart sync with your harvester whenever you need it. This technology allows you to command an operator-less tractor with a grain cart to drive to the combine, match the speed of the combine while the combine is unloading grain into the cart; then returning the tractor and grain cart to a designated parking location. This all happens while you keep on harvesting.
Smart sensors and internet of things (IoT)
The integration of AI with IoT and smart sensor technologies has paved the way for real-time monitoring and automation in agriculture. Smart sensors are deployed in fields and agricultural machines collect data on moisture levels, temperature, humidity, and nutrient content, among other parameters. AI algorithms process this data to provide producers with precise information about crop health, enabling early detection of diseases, pests and nutrient deficiencies. By leveraging this data, producers can take proactive measures to prevent crop losses and optimise resource usage before harvesting.
Computer vision techniques (like the CNH’s Augmenta technology) analyse images captured by cameras installed on agricultural machines to detect anomalies, such as weed growth, nutrient deficiencies or crop diseases. Machine learning algorithms can identify patterns and provide predictive analytics, as well as do adjustments in real time on spraying applications of herbicides and foliar feeding – thus helping producers to optimise the use of agricultural chemicals and to kerb pollution. Computer vision technology (VSN) is also used in visual guidance of agricultural machinery to optimise driving paths to lower the crop damage caused by trampling (Photo 1).
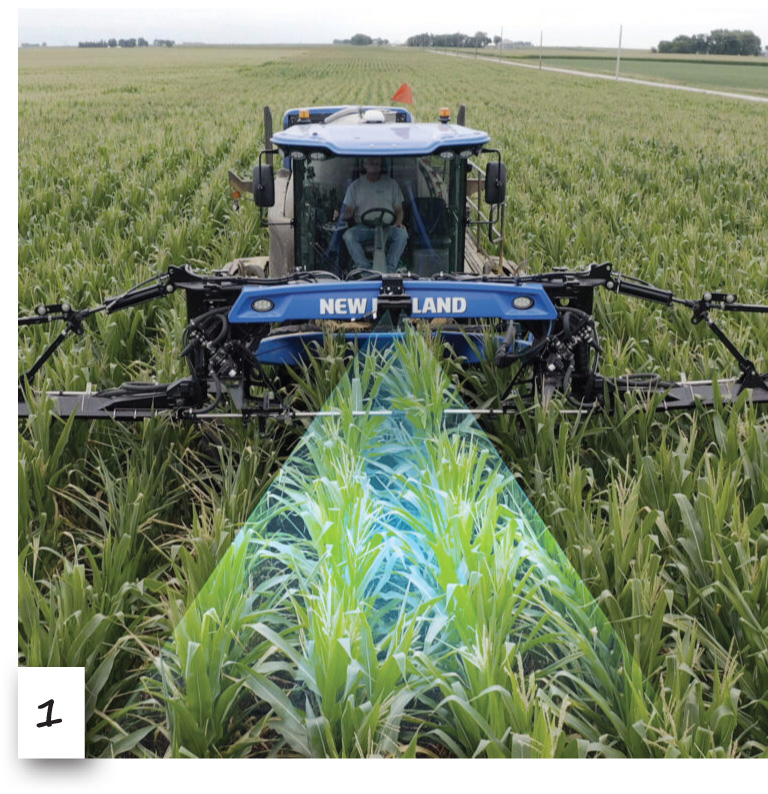
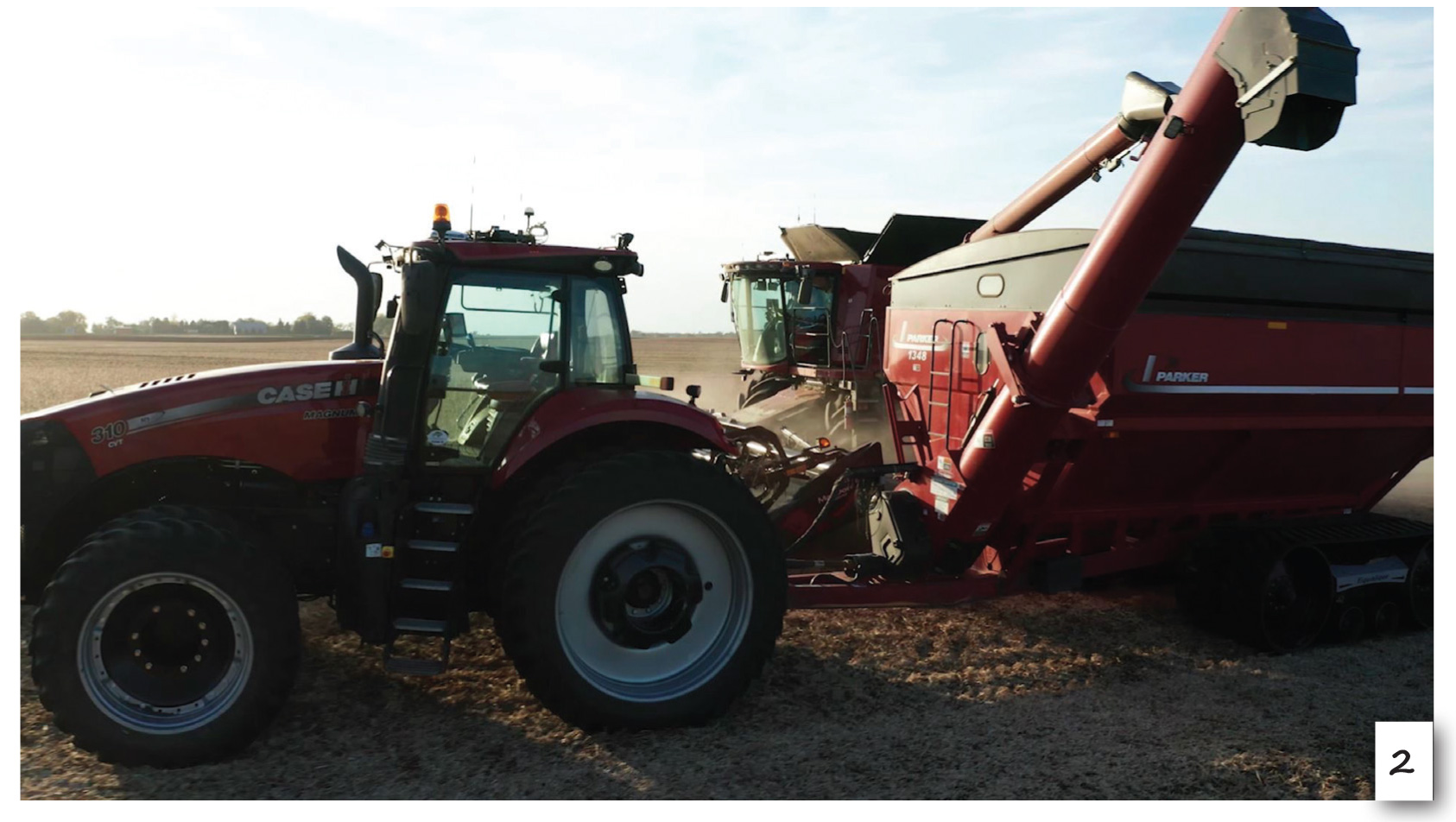
Sensors and AI algorithms on combines provide producers with the ability to optimise harvesting in real time whereby the combine bases an optimised decision on 280 000 000 data entries every 20 seconds with Harvest Command. The AI algorithm calculates with the sensor data of the combine to optimise the throughput to enable the grain quality and machine efficiency based on the parameters set by the operator. Class 8 combines from Case IH with Harvest Command use this technology to optimally adjust
settings of the components in the combine while harvesting for effective material throughput, adjusting the combine ground speed depending on the volume and moisture content of plant material, and cleaning the grain for a high quality and clean product.
Agricultural drones and robotics
Unmanned aerial vehicles (commonly known as drones), equipped with AI and computer vision capabilities, have become valuable tools for modern agriculture. Drones can survey large production areas quickly and efficiently, capturing high-resolution images and multispectral data. AI algorithms process these images to identify crop stress, plant diseases, or irregularities in growth patterns. This enables producers to target specific areas for intervention, reducing the need for manual scouting and improving the effectiveness of interventions. In the sugar cane agriculture industry, agricultural drones are used to survey planned production areas of which the data are used to produce 3D terrain models.
With the use of AI algorithms, it provides optimum rows of the longest length and slope efficiency. These rows are used to create guidance lines which are transferred to the tractors and combines for establishment and harvesting. The precise guidance on the sugar cane combine enables it to circumvent a great deal of trampling, which prolongs the productive life of the sugar cane plants. The longer rows increase the machine and fuel efficiency by shortening the unproductive turnaround times. This is more economical, lowers the production costs and increases the productivity per production unit.
Robotic technologies powered by AI are also making significant strides in agriculture. Autonomous robots equipped with sensors and computer vision systems can perform tasks such as seeding, weeding, harvesting, and sorting crops. These robots minimise the need for human labour and increase operational efficiency, particularly in labour-intensive processes like harvesting. AI algorithms enable robots to recognise and handle different crops with precision, resulting in reduced waste and increased productivity.
AI and control room management
Control rooms use AI, data mining and analytics based on the machine data to provide preventative maintenance guidance and usage methodologies for the optimal use of the equipment. This guidelines and alerts provide cost savings in various fields like repairs and fuel usage and in some instances prolong the useful life of equipment and wear parts. The equipment receives updated software or firmware over the air (OTA) with upgraded software and machine parameters which will provide the operator with new functionality and guidance. In high alert critical situations, the vehicle will advise the operator to stop the vehicle and request technical assistance. Notifications on issues on vehicles are also being sent to the technical staff of dealers in the preferred area of the client. This functionality prevents costly repairs to a certain extent.
Conclusion
The integration of AI in agriculture and agricultural equipment has the potential to transform the industry, making farming more efficient, sustainable, and profitable. By leveraging advanced technologies such as machine learning, predictive analytics, IoT, and robotics, producers can make more informed decisions, optimise resource allocation, and enhance productivity while reducing the environmental impact. The adoption of AI in agriculture can address key challenges facing the sector, ensuring sustainable food production and improving global food security. The interconnected systems and equipment with readily available data enable and promote the analytics with the use of AI to provide information for today and the future. As AI continues to advance, it is essential to invest in research, infrastructure, equipment, and training to enable its widespread adoption and unlock its full potential in shaping the future of farming.